Nowadays, people are used to post opinions and comment on others on social media. Compared to traditional data collection methods, such as questionnaires, polls, and phone interviews, online social media offers real-time information that can be utilized as an efficient yet effective way to study public stances on various issues. Therefore, automatically identifying user’s stance towards a target of concern using social media information has become a new trend in opinion mining.
In this Computational Social Science Workshop, Dr. Yupeng Li from the Department of Interactive Media of Hong Kong Baptist University shared his recent study on machine learning aided stance detection using user-generated contents and user interactions, respectively. COVID-19 vaccination is used as a sample target in his studies. First, Dr. Li introduced a newly constructed dataset that contains annotations of stances and the structures of conversation threads among the instances based on six major social media platforms in Hong Kong, and proposed a model termed BranchBERT that incorporates contextual information in conversation threads to detect user stances, e.g., support, against, or neutral. A new improvement–Conversational Multi-head Attention method was introduced as well, which tunes up the performance of BranchBERT model and can be implemented in both Chinese and English context.
Many users did not reveal their stances in their posts/comments or even did not post any contents. Their stances were typically embedded in their interactions with other users. To identify such implicit stances, Dr. Li presented a new graph neural network-based solution, Graph Neural Network (GNN)-based Stance Detection, and demonstrated its performance with a carefully constructed dataset, C19-VS, that consists of a large-scale interaction graph on vaccination stances from Twitter. To our best knowledge, it is the first implementation of stance detection using GNN.
Dr. Li’s work can help the policy makers make better use of the online social platform data to estimate and understand the real-time trend of the public stance towards vaccination, and can shed light on the framework of response to crises via social media analysis.
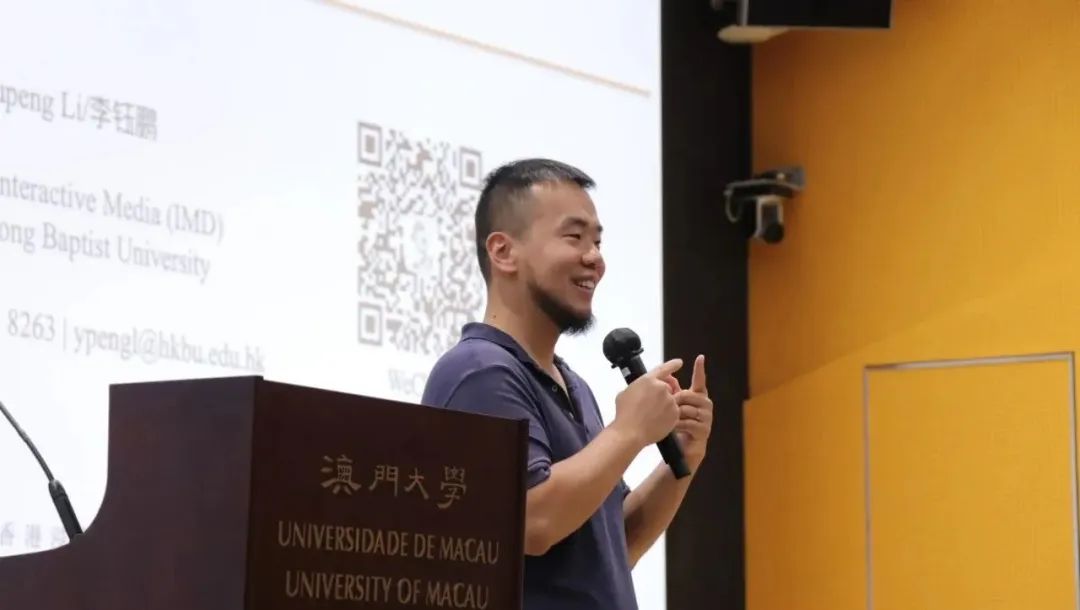
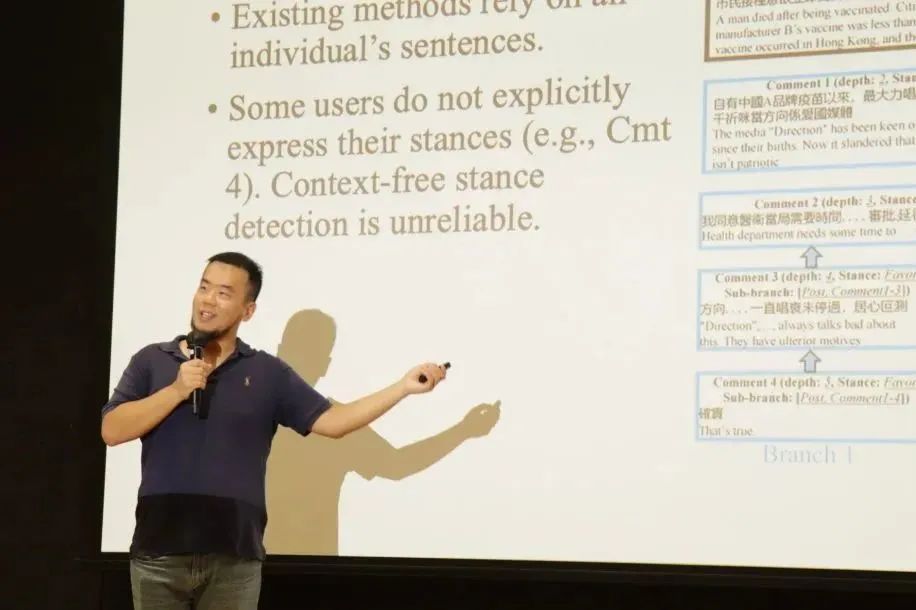
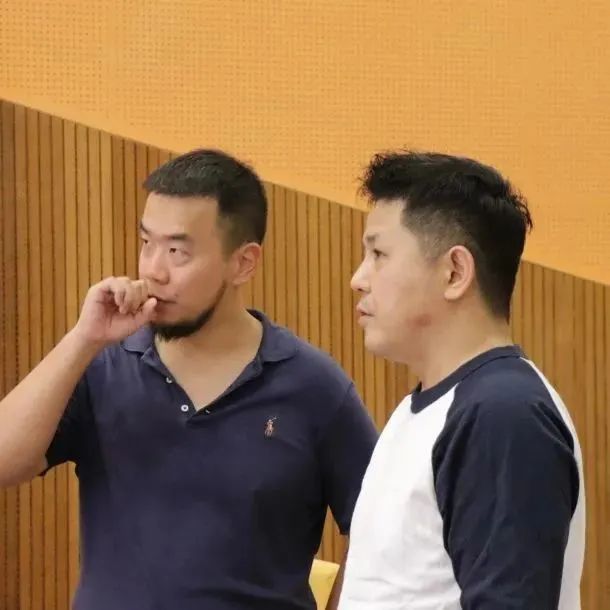
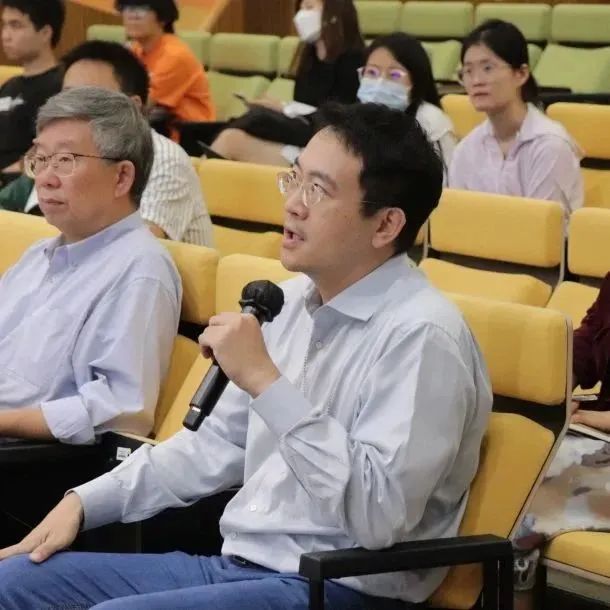
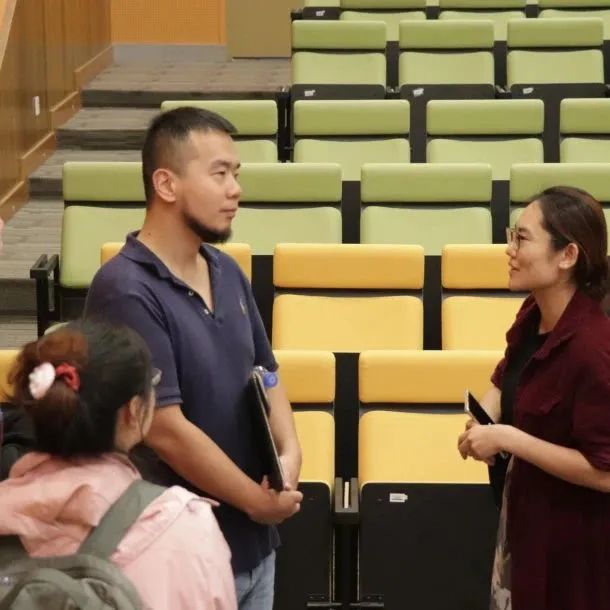
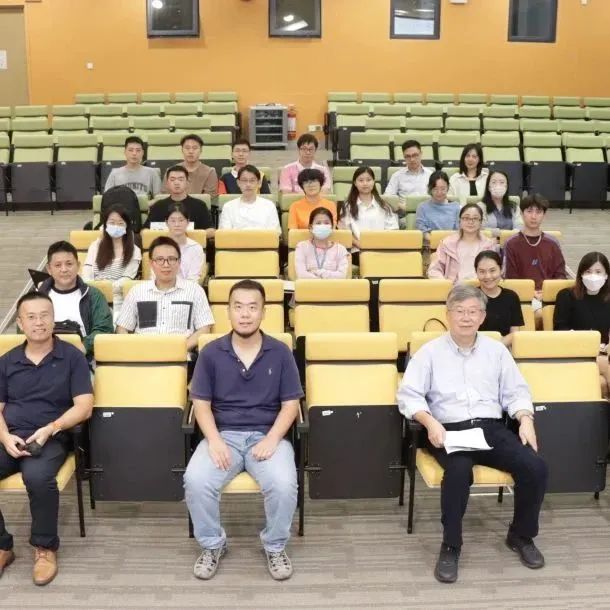